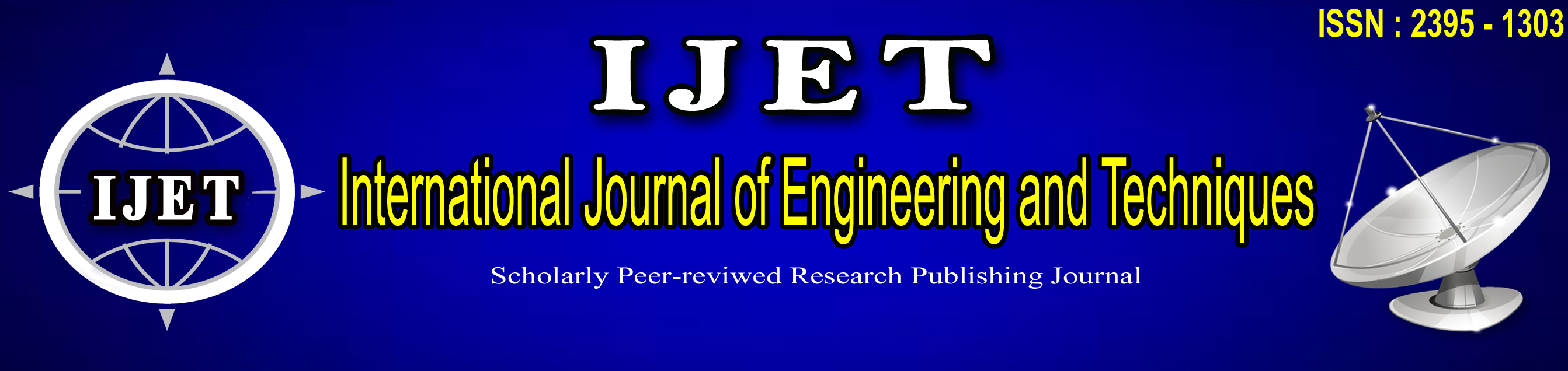
Submit your paper : editorIJETjournal@gmail.com ![]() ISSN : 2395-1303 Year of Publication : 2022 ![]() ![]() ![]() MLA Style: -P. Gayatri, Vakala Naga Sindhu, Modadugu Dimple Abhinaya Sri, Rayaluri Yagnitha STUDENT PERFORMANCE ANALYSIS AND PREDICTION USING MACHINE LEARNING , Volume 8 - Issue 6 November - December 2022 International Journal of Engineering and Techniques (IJET) ,ISSN:2395-1303 , www.ijetjournal.org APA Style: -P. Gayatri, Vakala Naga Sindhu, Modadugu Dimple Abhinaya Sri, Rayaluri Yagnitha STUDENT PERFORMANCE ANALYSIS AND PREDICTION USING MACHINE LEARNING , Volume 8 - Issue 6 November - December 2022 International Journal of Engineering and Techniques (IJET) ,ISSN:2395-1303 , www.ijetjournal.org Abstract Students have to fulfil the academic goals when they are studying in universities and colleges.They play an important role in achieving educational goals for colleges or themselves. Their intelligence is tested through various examinations, activities, attitude towards studies etc. So they should have an idea on how they should study accordingly. It gives them their analysis based on previous records so that they can know how much should they study for the coming examinations. The main goal of this project is to manage the progress of the students in their academics. Students will be provided their login credentials and using these login credentials they enter into their account. This mode helps them to reach their goals and ultimate targets by predicting their next scores precisely so that the students can work according to their estimated scores. Students get notifications to give a reminder to the students to reach their short-term goals. This also helps students by providing them with their subject-related best articles and YouTube links, which saves time for the student. This gives them a curiosity to learn and get clear and clean knowledge about the topic they learn. And further, we will keep on adding modules to improve our website according to the student's requirements. So, the proposed system helps students to reach their educational goals like getting good aggregates and they can analyse themselves properly. Based on the previous semester's grades he gets an idea of how to prepare for the next semester. Reference [1] J. Xu, K. H. Moon, and M. Van Der Schaar, “A Machine Learning Approach for Tracking and Predicting Student Performance in Degree Programs,” IEEE J. Sel. Top. Signal Process., vol. 11, no. 5, pp. 742–753, 2017. [2] K. P. Shaleena and S. Paul, “Data mining techniques for predicting student performance,” in ICETECH 2015 - 2015 IEEE International Conference on Engineering and Technology, 2015, no. March, pp. 0–2. [3] A. M. Shahiri, W. Husain, and N. A. Rashid, “A Review on Predicting Student’s Performance Using Data Mining Techniques,” in Procedia Computer Science, 2015. [4] P. M. Arsad, N. Buniyamin, and J. L. A. Manan, “A neural network students’ performance prediction model (NNSPPM),” 2013 IEEE Int. Conf. Smart Instrumentation, Meas. Appl. ICSIMA 2013, no. July 2006, pp. 26–27, 2013. [5] K. F. Li, D. Rusk, and F. Song, “Predicting student academic performance,” Proc. – 2013 7th Int. Conf. Complex, Intelligent, Softw. Intensive Syst. CISIS 2013, pp. 27–33, 2013. [6] Z. . Alharbi, J. . Cornford, L. .Dolder, and B. . De La Iglesia, “Using data mining techniques to predict students at risk of poor performance,” Proc. 2016 SAI Comput. Conf. SAI 2016, pp. 523–531, 2016. [7] I. E. Livieris, K. Drakopoulou, and P. Pintelas, “Predicting students’ performance using artificial neural networks,” Proc. 8th Pan-Hellenic Conf. “Information Commun. Technol.Educ., pp. 28–30, 2012. [8] H.Hamsa, S. Indiradevi, and J. J. Kizhakkethottam, “Student Academic Performance Prediction Model Using Decision Tree and Fuzzy Genetic Algorithm,” Procedia Technol., 2016. [9] P. MohdArsad, N. Buniyamin, and J. L. Ab Manan, “Neural Network and Linear Regression methods for prediction of students’ academic achievement,” IEEE Glob. Eng. Educ. Conf. EDUCON, no. April, pp. 916–921, 2014. [10] F. Sarker, T. Tiropanis, and H. C. Davis, “Linked data, data mining and external open data for better prediction of at-risk students,” in Proceedings - 2014 International Conference on Control, Decision and Information Technologies, CoDIT 2014, 2014. Keywords — credentials, performance, management system, academics, modules |