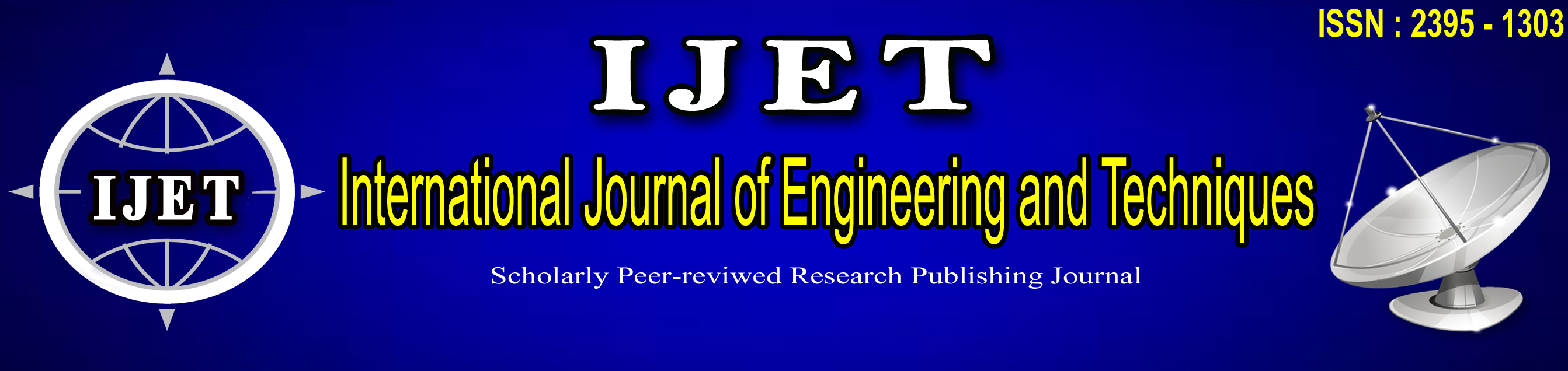
Submit your paper : editorIJETjournal@gmail.com ![]() ISSN : 2395-1303 Year of Publication : 2022 ![]() ![]() ![]() MLA Style: -Dr. Subba Reddy Borra, Neela Shanvitha, Anusha Tenneti, Ogirala Rajeswari IDENTIFICATION AND CHARACTERIZATION OF CYBERBULLYING DYNAMICS IN AN ONLINE SOCIAL NETWORK , Volume 8 - Issue 6 November - December 2022 International Journal of Engineering and Techniques (IJET) ,ISSN:2395-1303 , www.ijetjournal.org APA Style: -Dr. Subba Reddy Borra, Neela Shanvitha, Anusha Tenneti, Ogirala Rajeswari IDENTIFICATION AND CHARACTERIZATION OF CYBERBULLYING DYNAMICS IN AN ONLINE SOCIAL NETWORK , Volume 8 - Issue 6 November - December 2022 International Journal of Engineering and Techniques (IJET) ,ISSN:2395-1303 , www.ijetjournal.org Abstract This research applies a social network perspective to the issue of cyber aggression, or cyberbullying, on the social media platform Twitter. Cyber aggression is particularly problematic because of its potential for anonymity, and the ease with which so many others can join the harassment of victims. Utilizing a comparative case study methodology, the authors examined thousands of Tweets to explore the use of denigrating slurs and insults contained in public tweets that target an individual’s gender, race, or sexual orientation. Findings indicate cyber aggression on Twitter to be extensive and often extremely offensive, with the potential for serious, deleterious consequences for its victims. The study examined a sample of 84 aggressive networks on Twitter and visualize several social networks of communication patterns that emanate from an initial, aggressive tweet. The authors identify six social roles that users can assume in the network, noting differences in these roles by demographic category. Serious ethical concerns pertain to this technological, social problem. Reference [1] J. Tang, C. Aggarwal, and H. Liu, “Recommendations in signed social networks,” in Proceedings of the International Conference on WWW, 2016, pp. 31–40. [2] D. Liben-Nowell and J. Kleinberg, “The link-prediction problem forsocial networks,” Proceedings of the ASIS&T, vol. 58, no. 7, pp. 1019–1031, 2007. [3] U. Brandes and D. Wagner, “Analysis and visualization of social networks,” in Graph drawing software, 2004, pp. 321–340. [4] X. Hu, J. Tang, H. Gao, and H. Liu, “Social spammer detection with sentiment information,” In Proceedings of IEEE ICDM, pp. 180––189, 2014. [5] E. E. Buckels, P. D. Trapnell, and D. L. Paulhus, Trolls just want tohave fun, 2014, pp. 67:97–102. [6] S. Kumar, F. Spezzano, and V. Subrahmanian, “Accurately detectingtrolls in slashdot zoo via decluttering,” in Proceedings of IEEE/ACM ASONAM, 2014, pp. 188–195. [7] J. W. Patchin and S. Hinduja, “2016 cyberbullying data,” 2017. [8] C. R. Center, https://cyberbullying.org/bullying-laws. [9] D. Cartwright and F. Harary, “Structural balance: a generalization of heider’s theory.” Psychological review, vol. 63, no. 5, p. 277, 1956. [10] J. Leskovec, D. Huttenlocher, and J. Kleinberg, “Signed networks insocial media,” in Proceedings of the SIGCHI CHI, 2010, pp. 1361–1370. [11] R. Plutchik, “A general psychoevolutionary theory of emotion,” in Theories of emotion, 1980, pp. 3–33. [12] W. Medhat, A. Hassan, and H. Korashy, “Sentiment analysis algorithmsand applications: A survey,” Proceedings of the Ain Shams engineering journal, vol. 5, no. 4, pp. 1093–1113, 2014. [13] L. Tang and H. Liu, “Community detection and mining in social media,” Synthesis lectures on data mining and knowledge discovery, vol. 2, no. 1, pp. 1–137, 2010. [14] S. Bhagat, G. Cormode, and S. Muthukrishnan, “Node classification insocial networks,” in Social network data analytics, 2011, pp. 115–148. [15] J. Tang, Y. Chang, C. Aggarwal, and H. Liu, “A survey of signed networkmining in social media,” In Proceedings of the ACM Comput. Surv., no. 3, pp. 42:1–42:37, 2016. [16] J. Kunegis, J. Preusse, and F. Schwagereit, “What is the added valueof Cochrane Database of Systematic Reviews, vol. 4, no. 4, pp. CD008958, 2014. negative links in online social networks?” in Proceedings of the International Conference on WWW, 2013, pp. 727–736. [17] Z. Wu, C. C. Aggarwal, and J. Sun, “The troll-trust model for ranking insigned networks,” in Proceedings of the ACM International Conference on WSDM, 2016, pp. 447–456. [18] R. Zhao, A. Zhou, and K. Mao, “Automatic detection of cyberbullying on social networks based on bullying features,” in Proceedings of the ICDCN, 2016. [19] V. K. Singh, Q. Huang, and P. K. Atrey, “Cyberbullying detection using probabilistic socio-textual information fusion,” In Proceedings of the IEEE/ACM ASONAM, pp. 884—-887, 2016. [20] H. Hosseinmardi, S. A. Mattson, R. I. Rafiq, R. Han, Q. Lv, and S. Mishra, “Detection of cyberbullying incidents on the instagram social network,” In Proceedings of the CoRR, 2015. [21] J.-M. Xu, X. Zhu, and A. Bellmore, “Fast learning for sentiment analysison bullying,” in Proceedings of the First International WISDOM, 2012, pp. 10:1–10:6. [22] A. C. Squicciarini, S. M. Rajtmajer, Y. Liu, and C. H. Griffin, “Identificationand characterization of cyberbullying dynamics in an online Social network,” in Proceedings of the IEEE/ACM ASONAM, 2015, pp.280–285. [23] P. Galan-Garcia, J. De La Puerta, C. G´omez, I. Santos, and P. Bringas, “Supervised machine learning for the detection of troll profiles in twitter social network: Application to a real case of cyberbullying,” vol. 24, pp. 42–53, 2014. [24] D. Chatzakou, N. Kourtellis, J. Blackburn, E. De Cristofaro, G. Stringhini,and A. Vakali, “Mean birds: Detecting aggression and bullying on Twitter,” in Proceedings of the ACM on WebSci, 2017, pp. 13–22. [25] L. Cheng, J. Li, Y. N. Silva, D. L. Hall, and H. Liu, “Xbully: Cyberbullying detection within a multi-modal context,” in Proceedingsof the Twelfth ACM International Conference on Web Search andDataMining, 2019, pp. 339–347. Keywords — IDENTIFICATION AND CHARACTERIZATION OF CYBERBULLYING DYNAMICS IN AN ONLINE SOCIAL NETWORK |