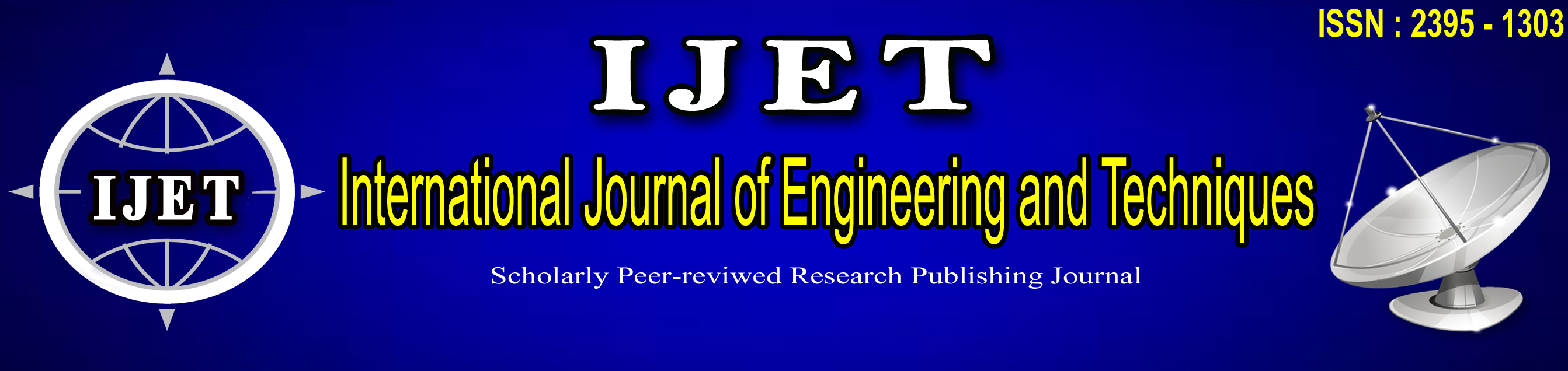
Submit your paper : editorIJETjournal@gmail.com ![]() ISSN : 2395-1303 Year of Publication : 2022 ![]() ![]() ![]() MLA Style: -Guntipally Shubhasri, Dr. G.N.R. Prasad VARIOUS SOFTWARE TEST CASES FOR COVID-19 POSITIVE CASES PREDICTION SYSTEM , Volume 8 - Issue 5 September - October 2022 International Journal of Engineering and Techniques (IJET) ,ISSN:2395-1303 , www.ijetjournal.org APA Style: -Guntipally Shubhasri, Dr. G.N.R. Prasad VARIOUS SOFTWARE TEST CASES FOR COVID-19 POSITIVE CASES PREDICTION SYSTEM , Volume 8 - Issue 5 September - October 2022 International Journal of Engineering and Techniques (IJET) ,ISSN:2395-1303 , www.ijetjournal.org Abstract In the process of developing software, testing is essential. Testing is a crucial and beneficial phase of the software development life cycle in every organisation. Software testing methodologies vary from firm to company. Testing software is not a simple process. There will be difficulties with both coding and decoding every single day. In order to enhance decision- making regarding the future course of action, machine learning (ML) based forecasting methods have demonstrated their usefulness to foresee in perioperative outcomes. The detection and prioritisation of negative aspects for a threat was a long-standing requirement in many application domains where ML models were deployed. Many prediction techniques are now in use to address forecasting issues. This study demonstrates how machine learning (ML) models may be used to forecast the number of COVID-19 patients who will be affected in the future, which is currently regarded to be a threat to mankind. Four widely used forecasting models, including support vector machine (SVM) and linear regression (LR), were used in this study to anticipate the potentially dangerous ingredients (SVM). Reference 1.S. Makridakis, E. Spiliotis, and V. Assimakopoulos, “Statistical and machine learning forecasting methods: Concerns and ways forward,” PloS one, vol. 13, no. 3, 2018. 2. G. Bontempi, S. B. Taieb, and Y.-A. Le Borgne, “Machine learning strategies for time series forecasting,” in European business intelligence summer school. Springer, 2012, pp. 62–77. 3. F. E. Harrell Jr, K. L. Lee, D. B. Matchar, and T. A. Reichert, “Regression models for prognostic prediction: advantages, problems, and suggested solutions.” Cancer treatment reports, vol. 69, no. 10, pp. 1071–1077, 1985. 4.P. Lapuerta, S. P. Azen, and L. LaBree, “Use of neural networks in predicting the risk of coronary artery disease,” Computers and Biomedical Research, vol. 28, no. 1, pp. 38–52, 1995. 5.K. M. Anderson, P. M. Odell, P. W. Wilson, and W. B. Kannel, “Cardiovascular disease risk profiles,” American heart journal, vol. 121, no. 1, pp. 293–298, 1991. 6. H. Asri, H. Mousannif, H. Al Moatassime, and T. Noel, “Using machine learning algorithms for breast cancer risk prediction and diagnosis,” Procedia Computer Science, vol. 83, pp. 1064–1069, 2016. 7. F. Petropoulos and S. Makridakis, “Forecasting the novel coronavirus covid-19,” Plos one, vol. 15, no. 3, p. e0231236, 2020. 8.G. Grasselli, A. Pesenti, and M. Cecconi, “Critical care utilization for the covid-19 outbreak in lombardy, italy: early experience and forecast during an emergency response,” Jama, 2020. 9. WHO. Naming the coronavirus disease (covid-19) and the virus that causes it. [Online]. Available: https://www.who.int/emergencies/diseases/novelcoronavirus-2019/technical- guidance/naming-the-coronavirus-disease- (covid-2019)-and-the-virus-that-causes-it 10.C. P. E. R. E. Novel et al., “The epidemiological characteristics of an outbreak of 2019 novel coronavirus diseases (covid-19) in china,” Zhonghua liu xing bing xue za zhi= Zhonghua liuxingbingxue zazhi, vol. 41, no. 2, p. 145, 2020. 11. Involve testing throughout the SDLC available at http://www.silverpath.com Keywords — Software Testing, Machine learning, Covid-19, Linear Regression, Support vector machine. |