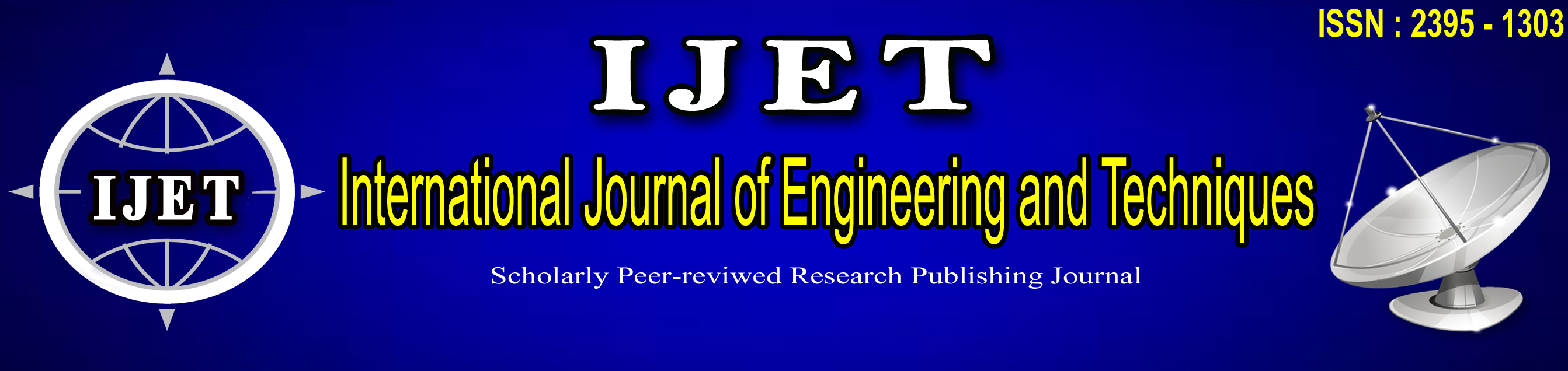
Submit your paper : editorIJETjournal@gmail.com ![]() ISSN : 2395-1303 Year of Publication : 2022 ![]() ![]() ![]() MLA Style: -Mr. P. DEVENDRA BABU, G.Supraja, D.Haripriya, E.Ravalika DETECTING BULIDING DAMAGE INTENSITY DURING DISASTERS USING MACHINE LEARNING , Volume 8 - Issue 5 September - October 2022 International Journal of Engineering and Techniques (IJET) ,ISSN:2395-1303 , www.ijetjournal.org APA Style: -Mr. P. DEVENDRA BABU, G.Supraja, D.Haripriya, E.Ravalika DETECTING BULIDING DAMAGE INTENSITY DURING DISASTERS USING MACHINE LEARNING , Volume 8 - Issue 5 September - October 2022 International Journal of Engineering and Techniques (IJET) ,ISSN:2395-1303 , www.ijetjournal.org Abstract Previous applications of machine learning in remote sensing for the identification of broken buildings within the aftermath of a large-scale disaster are no-hit. However, normal ways don't take into account the complexness and prices of compilation a coaching knowledge set when a large-scale disaster. during this article, we tend to study disaster events within which the intensity is sculpturesque via numerical simulation and/or instrumentation. For such cases, 2 absolutely automatic procedures for the detection of severely broken buildings ar introduced. the basic assumption is that samples that ar placed in areas with low disaster intensity primarily represent nondamaged buildings. moreover, areas with moderate to robust disaster intensities probably contain broken and nondamaged buildings. below this assumption, a procedure that's supported the automated choice of coaching samples for learning and calibrating the quality support vector machine classifier is employed. The second procedure is predicated on the utilization of 2 regularization parameters to outline the support vectors. These frameworks avoid the gathering of labeled building samples via field surveys and/or visual examination of optical pictures, which needs a major quantity of your time. Reference [1] B. Pan, Z. Shi, and X. Xu, “Multi objective-based sparse representation classifier for hyperspectral imagery using limited samples,” IEEE Trans. Geosci. Remote Sens., vol. 57,no. 1, pp. 239–249, Jan. 2019. [2] C. Geiß, P. A. Pelizari, L. Blickensdörfer, and H. Taubenböck, “Virtualsupport vector machines with self- learning strategy for classification of multispectral remote sensing imagery,” ISPRS J. Photogramm. RemoteSens., vol. 151, pp. 42– 58, May 2019. C. Geiß, M. Thoma, M. Pittore, M. Wieland, S. W. Dech, and H. Taubenbock, “Multitask active learning for characterization of built environments with multisensor Earth observation data,” IEEE J. Sel. TopicsAppl. Earth Observ. Remote Sens., vol. 10, no. 12, pp. 5583–5597, Dec. 2017. C. Persello, A. Boularias, M. Dalponte, T. Gobakken, E. Næsset, and B. Schölkopf, “Cost-sensitive active learning with lookahead: Optimizingfield surveys for remote sensing data classification,” IEEE Trans. Geosci. Remote Sens., vol. 52, no. 10, pp. 6652–6664, Oct. 2014. [3] C. Geib, M. Thoma, and H. Taubenbock, “Cost- sensitive multitask active learning for characterization of urban environments with remote sensing,” IEEE Geosci. Remote Sens. Lett., vol. 15, no. 6, pp. 922– 926,Jun. 2018. [4] C. Huang et al., “Use of a dark object concept and support vectormachines to automate forest cover change analysis,” Remote Sens. Environ., vol. 112, no. 3, pp. 970–985, Mar. 2008. [5] C. Geiß, P. A. Pelizari, S. Bauer, A. Schmitt, and H. Taubenböck, “Automatic trainingset compilation with multisource geodata for DTMgeneration from the TanDEM-X DSM,”IEEEGeosci. Remote Sens. Lett., vol. 17, no. 3, pp. 456–460, Mar. 2019. [6] E. Booth, K. Saito, R. Spence, G. Madabhushi, and R. T. Eguchi, “Validating assessments of seismic damage made from remote sensing,”Earthq. Spectra, vol. 27, no. 1,pp. 157– 177, Oct. 2011. Keywords — DETECTING BULIDING DAMAGE INTENSITY DURING DISASTERS USING MACHINE LEARNING |