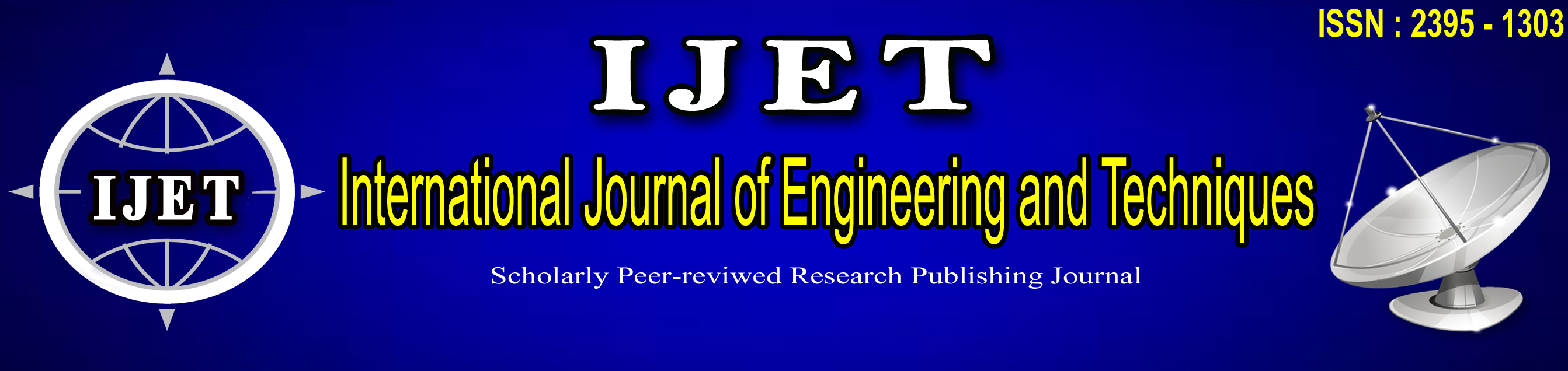
Submit your paper : editorIJETjournal@gmail.com ![]() ISSN : 2395-1303 Year of Publication : 2022 ![]() ![]() ![]() MLA Style: -Mr. M.Sunil Kumar, M.Sravani, P.Poojitha, Pranavi joshi MACHINE LEARNING FRAMEWORK FOR DETECTING SPAMMER AND FAKE USERS , Volume 8 - Issue 5 September - October 2022 International Journal of Engineering and Techniques (IJET) ,ISSN:2395-1303 , www.ijetjournal.org APA Style: -Mr. M.Sunil Kumar, M.Sravani, P.Poojitha, Pranavi joshi MACHINE LEARNING FRAMEWORK FOR DETECTING SPAMMER AND FAKE USERS , Volume 8 - Issue 5 September - October 2022 International Journal of Engineering and Techniques (IJET) ,ISSN:2395-1303 , www.ijetjournal.org Abstract Social networking sites engage millions of users around the world. The users’ interactions with these social sites, such as Twitter and Facebook have a tremendous impact and occasionally undesirable repercussions for the daily life. The prominent social networking sites have turned into atarget platform for the spammers to disperse a huge amount of irrelevant and deleterious information. Twitter, for example, has become one of the most extravagantly used platforms of all times and therefore allows an unreasonable amount of spamming. Fake users send undesired tweets to users to promote services or websites that not only affect the legitimate users but also disrupt the resource consumption. Moreover, the possibility of expanding invalid information to users through fake identities has increased that results in the unrolling of harmful content. Recently, the detection of spammers and identification of fake users on Twitter has become a common area of research in contemporary online social Networks (OSNs). In this paper, we perform a review of techniques usedfor detecting spammers on Twitter. Moreover, a taxonomy of the Twitter spam detection approachesis presented that classifies the techniques based on their ability to detect: (i) fake content, (ii) spam based on URL, (iii) spam in trending topics, and (iv) fake users. The presented techniques are also compared based on various features, such as user features, content features, graph features, structure features, and time features. We are hopeful that the presented study will be a useful resource for researchers to find the highlights of recent developments in Twitter spam detection on a single platform Reference [1] B. Erçahin, Ö. Akta³, D. Kilinç, and C. Akyol, ``Twitter fake account detection,'' in Proc. Int. Conf. Comput. Sci. Eng. (UBMK), Oct. 2017, pp. 388392. [2] F. Benevenuto, G. Magno, T. Rodrigues, and V. Almeida, ``Detecting spammers on Twitter,'' in Proc. Collaboration, Electron. Messaging, AntiAbuse Spam Conf. (CEAS), vol. 6, Jul. 2010, p. 12. [3] S. Gharge, and M. Chavan, ``An integrated approach for malicious tweets detection using NLP,'' in Proc. Int. Conf. Inventive Commun. Comput. Technol. (ICICCT), Mar. 2017, pp. 435438. [4] T. Wu, S. Wen, Y. Xiang, and W. Zhou, ``Twitter spam detection: Survey of new approaches and comparative study,'' Comput. Secur., vol. 76, pp. 265284, Jul. 2018. [5] S. J. Soman, ``A survey on behaviors exhibited by spammers in popular social media networks,'' in Proc. Int. Conf. Circuit, Power Comput. Technol. (ICCPCT), Mar. 2016, pp. 16. [6] A. Gupta, H. Lamba, and P. Kumaraguru, ``1.00 per RT #BostonMarathon # prayforboston: Analyzing fake content on Twitter,'' in Proc. eCrime Researchers Summit (eCRS), 2013, pp. 112 Keywords — MACHINE LEARNING FRAMEWORK FOR DETECTING SPAMMER AND FAKE USERS |