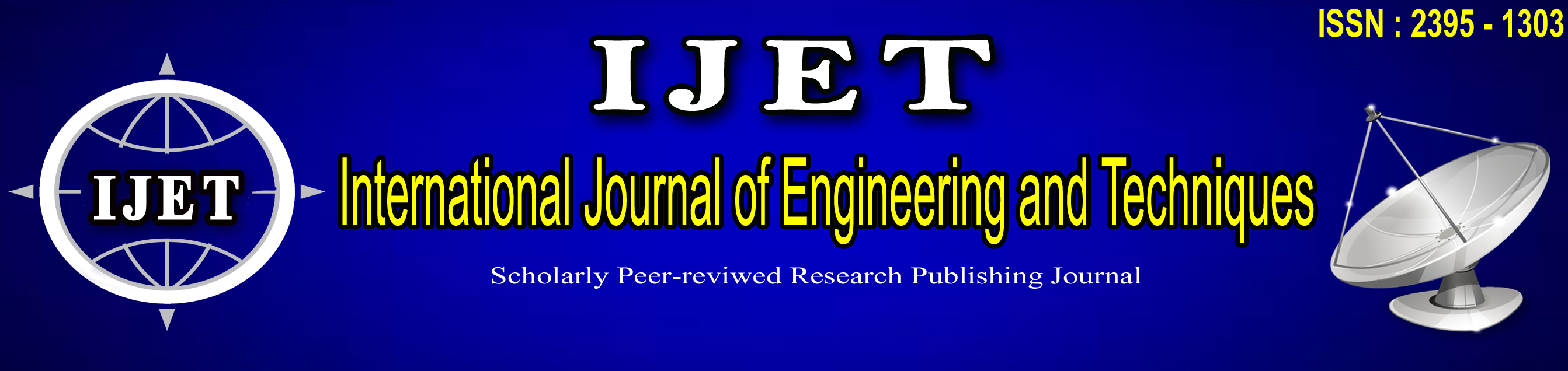
Submit your paper : editorIJETjournal@gmail.com ![]() ISSN : 2395-1303 Year of Publication : 2022 ![]() ![]() ![]() MLA Style: -B.Durga Bhavani ,V.Sahithi ,S.Sai Priya .,R. Aishwarya PRIVACY PRESERVING SOCIAL MEDIA DATA PUBLISHING FOR PERSONALIZED RANKING BASED RECOMMENDATION , Volume 8 - Issue 5 September - October 2022 International Journal of Engineering and Techniques (IJET) ,ISSN:2395-1303 , www.ijetjournal.org APA Style: -B.Durga Bhavani ,V.Sahithi ,S.Sai Priya .,R. Aishwarya PRIVACY PRESERVING SOCIAL MEDIA DATA PUBLISHING FOR PERSONALIZED RANKING BASED RECOMMENDATION , Volume 8 - Issue 5 September - October 2022 International Journal of Engineering and Techniques (IJET) ,ISSN:2395-1303 , www.ijetjournal.org Abstract A personalised recommendation is essential to assisting users in finding relevant information. To mine user preferences, it frequently uses a sizable collection of user data, particularly information about users' online behaviour (such as tagging, rating, and check-ins on social media). While private information (such as gender) may frequently be deduced from user activity data, exposing such data exposes users to inference attacks. In this research, we introduced Priv Rank, a continuous privacy- preserving social media data publishing architecture that allows for tailored ranking-based recommendations while defending users against inference assaults. Its main concept is to continuously obfuscate user activity data in order to limit the privacy leakage of user-specified private data within a certain data distortion budget. This also limits the ranking loss brought on by the data obfuscation.process in order to preserve the utility of the data for enabling recommendations. Reference [1] S. Salamatian, A. Zhang, F. du Pin Calmon, S. Bhamidipati, N. Fawaz, B. Kveton, P. Oliveira, and N. Taft, “How to hide the elephant-or the donkey-in the room: Practical privacy against statistical inference for large data,” in Proc. of GlobalSIP. IEEE, 2013. [2] D. Yang, D. Zhang, Q. Bingqing, and P. Cudre- Mauroux, “Privcheck: Privacy- preserving check-in data publishing for personalized location-based services,” in Proc. of UbiComp’16. ACM, 2016. [3] C. Li, H. Shirani-Mehr, and X. Yang, “Protecting individual information against inference attacks in data publishing,” in Advances in Databases: Concepts, Systems and Applications. Springer, 2007, pp. 422–433. [4] B. Fung, K. Wang, R. Chen, and P. S. Yu, “Privacy- preserving data publishing: A survey of recent developments,” ACM Computer Survey, vol. 42, no. 4, p. 14, 2010. [5] I. A. Junglas, N. A. Johnson, and C. Spitzm ¨ uller, “Personality traits and concern for privacy: an empirical study in the context of location-based services,” European Journal of Information Systems, vol. 17, no. 4, pp. 387– 402, 2008. [6] P. Cremonesi, Y. Koren, and R. Turrin, “Performance of recommender algorithms on top-n recommendation tasks,” in Proc. of RecSys’10. ACM, 2010, pp. 39–46. [7] N. Li, R. Jin, and Z.-H. Zhou, “Top rank optimization in linear time,” in Advances in neural information processing systems, 2014,pp. 1502–1510. [8] M. G. Kendall, “Rank correlation methods.” 1948. [9] L. Sweeney, “k-anonymity: A model for protecting privacy,” International Journal of Uncertainty, Fuzziness and Knowledge-Based [10] L. Sankar, S. R. Rajagopalan, and H. V. Poor, “Utility-privacy tradeoffs in databases: An information- theoretic approach,” IEEE Transactions on Information Forensics and Security, vol. 8, no. 6, pp.838–852, 2013. [11] A. Machanavajjhala, D. Kifer, J. Gehrke, and M. Venkitasubramaniam,“l-diversity: Privacy beyond k-anonymity,” ACM Transactions on Knowledge Discovery from Data, vol. 1, no. 1, p. 3, 2007. [12] C. Dwork, “Differential privacy,” in Automata, languages and programming.Springer, 2006, pp. 1–12. [13]F. du Pin Calmon and N. Fawaz, “Privacy against statistical inference,” in Proc. of Allerton’12. IEEE, 2012, pp. 1401–1408. [14]A. Zhang, S. Bhamidipati, N. Fawaz, and B. Kveton, “Priview: Media consumption and recommendation meet privacy againstinference attacks,” IEEE Web, vol. 2, 2014. [15]S. Salamatian, A. Zhang, F. du Pin Calmon, S. Bhamidipati, N. Fawaz, B. Kveton, P. Oliveira, and N. Taft, “Managing your private and public data: Bringing down inference attacks against your privacy,” IEEE Journal of Selected Topics in Signal Processing,vol. 9, no. 7, pp. 1240–1255, 2015. [16]W. Chen, T.-Y. Liu, Y. Lan, Z.-M. Ma, and H. Li, “Ranking measuresand loss functions in learning to rank,” in Proc. of NIPS, 2009, pp. 315–323. [17]M. B. Eisen, P. T. Spellman, P. O. Brown, and D. Botstein, “Clusteranalysis and display of genome-wide expression patterns,” PNAS, vol. 95, no. 25, pp. 14 863–14 868, 1998. [18] R. Baeza -Yates, B. Ribeiro-Neto et al., Modern information retrieval ACM press New York, 1999, vol. 463. Keywords — PRIVACY PRESERVING SOCIAL MEDIA DATA PUBLISHING FOR PERSONALIZED RANKING BASED RECOMMENDATION |