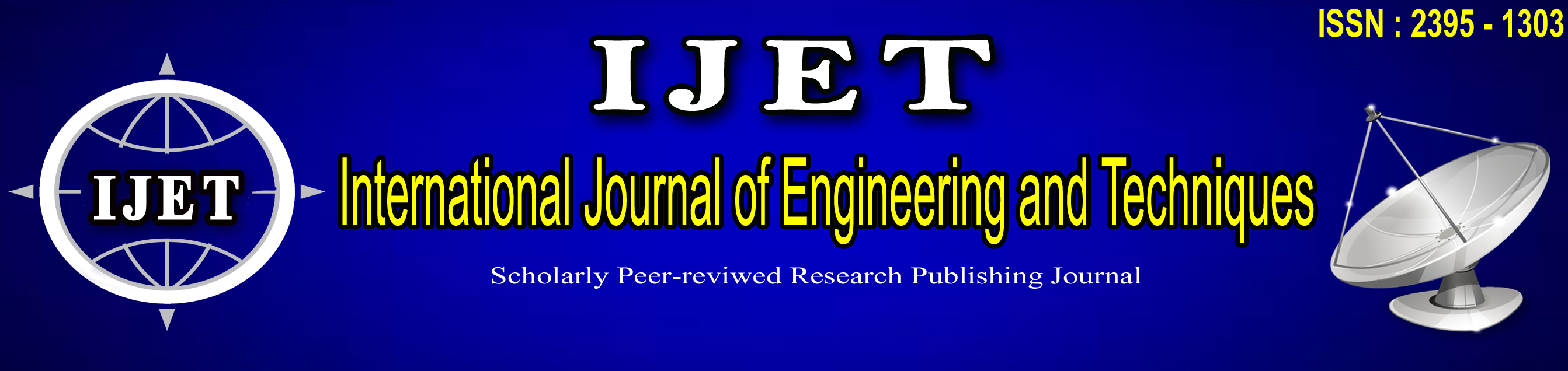
Submit your paper : editorIJETjournal@gmail.com ![]() ISSN : 2395-1303 Year of Publication : 2022 ![]() ![]() ![]() MLA Style: -Jayarajan S, Bhavana.K, Swathika.K ,Laya M Predicting Power Utilization In Large Vehicles Using ML , Volume 8 - Issue 5 September - October 2022 International Journal of Engineering and Techniques (IJET) ,ISSN:2395-1303 , www.ijetjournal.org APA Style: -Jayarajan S, Bhavana.K, Swathika.K ,Laya M Predicting Power Utilization In Large Vehicles Using ML , Volume 8 - Issue 5 September - October 2022 International Journal of Engineering and Techniques (IJET) ,ISSN:2395-1303 , www.ijetjournal.org Abstract Instead of the conventional time period, we employed the vehicle trip distance while creating customized machine learning models for fuel use. Seven predictors generated from vehicle speed and road grade are combined with this method to create a highly predictive neural network model for average fuel consumption in large vehicles. To reduce fuel usage across the board, the suggested methodology can be quickly established and implemented for each individual vehicle in a fleet. The model's predictors are combined over predetermined window sizes for distance travelled. The evaluation of various window widths reveals that a 1 km window has a 0.91 coefficient of determination and a mean absolute peak-to-peak percent error that is less. Reference [1]B. Lee, L. Quinones, and J. Sanchez, “Development of greenhouse gas emissions model for 2014-2017 heavy-and medium-duty vehicle compliance,” SAE Technical Paper, Tech. Rep., 2011. [2] G. Fontaras, R. Luz, K. Anagnostopoulus, D. Savvidis, S. Hausberger, and M. Rexeis, “Monitoring co2 emissions from hdv in europe-an methodolgical approach,” in 20th International Transport and Air Pollution Conference, 2014. [3] S. Wickramanayake and H. D. Bandara, “Fuel consumption prediction of fleet vehicles using machine learning: A comparative study,” in Moratuwa Engineering Research Conference (MERCon), 2016. IEEE, 2016, pp. 90–95. [4] L. Wang, A. Duran, J. Gonder, and K. Kelly, “Modeling heavy/mediumduty fuel consumption based on drive cycle properties,” SAE Technical Paper, Tech. Rep., 2015. [5] Fuel Economy and Greenhouse gas exhaust emissions of motor vehicles Subpart B - Fuel Economy and Carbon-Related Exhaust Emission Test Procedures, Code of Federal Regulations Std. 600.111-08, Apr 2014. [6] SAE International Surface Vehicle Recommended Practice, Fuel Consumption Test Procedure - Type II, Society of Automotive Engineers Std., 2012. [7] F. Perrotta, T. Parry, and L. C. Neves, “Application of machine learning for fuel consumption modelling of trucks,” in Big Data (Big Data), 2017 IEEE International Conference on. IEEE, 2017, pp. 3810–3815. [8] S. F. Haggis, T. A. Hansen, K. D. Hicks, R. G. Richards, and R. Marx, “In-use evaluation of fuel economy and emissions from coal haul trucks using modified sae j1321 procedures and pems,” SAE International Journal of Commercial Vehicles, vol. 1, no. 2008-01-1302, pp. 210–221, 2008. [9] A. Ivanco, R. Johri, and Z. Filipi, “Assessing the regeneration potential for a refuse truck over a real-world duty cycle,” SAE International Journal of Commercial Vehicles, vol. 5, no. 2012-01-1030, pp. 364–370, 2012. [10] A. A. Zaidi, B. Kulcsr, and H. Wymeersch, “Back-pressure traffic signal control with fixed and adaptive routing for urban vehicular networks,” IEEE Transactions on Intelligent Transportation Systems, vol. 17, no. 8, pp. 2134–2143, Aug 2016. [11] J. Zhao, W. Li, J. Wang, and X. Ban, “Dynamic traffic signal timing optimization strategy incorporating various vehicle fuel consumption characteristics,” IEEE Transactions on Vehicular. Keywords — Predicting Power Utilization In Large Vehicles Using ML |