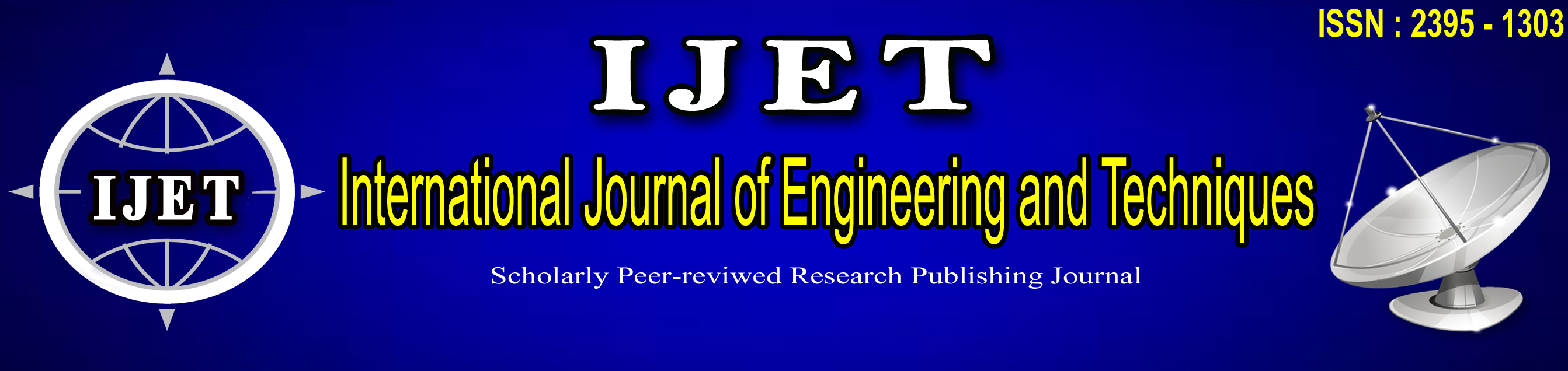
Submit your paper : editorIJETjournal@gmail.com ![]() ISSN : 2395-1303 Year of Publication : 2022 ![]() ![]() ![]() MLA Style: -Sushma G, Chinmayee M,Sindhu K Agriculture Crop Recommendations Based on Productivity and Season , Volume 8 - Issue 5 September - October 2022 International Journal of Engineering and Techniques (IJET) ,ISSN:2395-1303 , www.ijetjournal.org APA Style: -Sushma G, Chinmayee M,Sindhu K Agriculture Crop Recommendations Based on Productivity and Season , Volume 8 - Issue 5 September - October 2022 International Journal of Engineering and Techniques (IJET) ,ISSN:2395-1303 , www.ijetjournal.org Abstract Due to the variable climatic factors of the environment, there is a necessity to have an efficient technique to facilitate the crop cultivation and to lend a hand to the farmers in their production and management. A system of recommendations can be provided to a farmer to help them in crop cultivation with the help of data mining. To implement such an approach, crops are recommended based on its climatic factors and quantity. Data Analytics paves a way to evolve useful extraction from agricultural databases. Crop Dataset has been analysed and recommendation of crops is done based on productivity and season. Reference [1] S. H. Pahus, A. T. Hansen, and A.-M. Hvas, “Thrombophilia testing in young patients with ischemic stroke,” Thrombosis research, vol. 137, pp. 108–112, 2016. [2] P. Govindarajan, R. K. Soundarapandian, A. H. Gandomi, R. Patan, P. Jayaraman, and R. Manikandan, “Classification of stroke disease using machine learning algorithms,” Neural Computing and Applications, pp. 1–12. [3] L. T. Kohn, J. Corrigan, M. S. Donaldson, et al., To err is human: building a safer health system, vol. 6. National academy press Washington, DC, 2000. [4] R. Jeena and S. Kumar, “Stroke prediction using svm,” in 2016 International Conference on Control, Instrumentation, Communication and Computational Technologies (ICCICCT), pp. 600– 602, IEEE, 2016. [5] P. A. Sandercock, M. Niewada, and A. Członkowska, “The international stroke trial database,” Trials, vol. 13, no. 1, pp. 1–1, 2012. [6] M. S. Singh and P. Choudhary, “Stroke prediction using artificial intelligence,” in 2017 8th Annual Industrial Automation and Electromechanical Engineering Conference (IEMECON), pp. 158–161, IEEE, 2017. [7] S. Y. Adam, A. Yousif, and M. B. Bashir, “Classification of ischemic stroke using machine learning algorithms,” Int J Comput Appl, vol. 149, no. 10, pp. 26–31, 2016. [8] A. Sudha, P. Gayathri, and N. Jaisankar, “Effective analysis and predictive model of stroke disease using classification methods,” International Journal of Computer Applications, vol. 43, no. 14, pp. 26– 31, 2012. [9] G. Kaur and A. Chhabra, “Improved j48 classification algorithm for the prediction of diabetes,” International Journal of Computer Applications, vol. 98, no. 22, 2014. [10] I. H. Witten, E. Frank, M. A. Hall, and C. J. Pal, Data Mining: Practical machine learning tools and techniques. Morgan Kaufmann, 2016. [11] P. Sewaiwar and K. K. Verma, “Comparative study of various decision tree classification algorithm using weka,” International Journal of Emerging Research in Management &Technology, vol. 4, pp. 2278– 9359, 2015. [12] K. A. Shakil, S. Anis, and M. Alam, “Dengue disease prediction using weka data mining tool,” arXiv preprint arXiv:1502.05167, 2015. Keywords — Machine learning: Dataset: Computer Vision. |