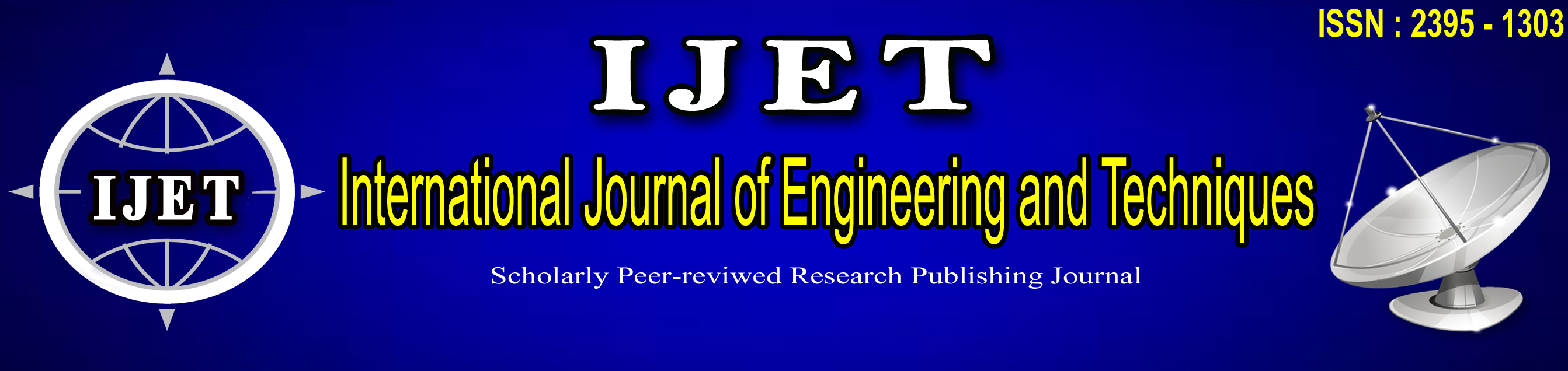
Submit your paper : editorIJETjournal@gmail.com ![]() ISSN : 2395-1303 Year of Publication : 2022 ![]() ![]() ![]() MLA Style: - Olorunnishola, A. A. G and Oladebeye, D. H , DEVELOPMENT OF A STATISTICAL MODEL TO PREDICT THE EFFECTS OF FAILURE AND PRODUCTION RATES ON ELECTRICAL ENERGY CONSUMPTION OF A LUBRICATING OIL INDUSTRY , Volume 8 - Issue 3 May - June 2022 International Journal of Engineering and Techniques (IJET) ,ISSN:2395-1303 , www.ijetjournal.org APA Style: - Olorunnishola, A. A. G and Oladebeye, D. H , DEVELOPMENT OF A STATISTICAL MODEL TO PREDICT THE EFFECTS OF FAILURE AND PRODUCTION RATES ON ELECTRICAL ENERGY CONSUMPTION OF A LUBRICATING OIL INDUSTRY , Volume 8 - Issue 3 May - June 2022 International Journal of Engineering and Techniques (IJET) ,ISSN:2395-1303 , www.ijetjournal.org Abstract This work presents formulation of linear regression model for the effect of energy consumption and production rates on the breakdowns of Lubcon oil production line. The model validation confirmed the existence of statistical relationships between breakdowns and energy consumption and production rates. Applying data collected from the Lubcon oil industry, R2 value of 98.6% was obtained for Lubcon oil production line model; thus, indicating that about 98.6% of the variation in electrical energy consumption could be explained by breakdowns and production rates, thus reducing the probability of consuming excess energy due to unplanned breakdowns in the production line to the barest minimum. The multiple linear regression models obtained by using the control and dependent variables give good estimation. The regression model also showed that given the failure (breakdowns) and production rates, the expected electrical energy consumption can be determined, thus, enabling the maintenance personnel to significantly monitor and reduce energy consumption in the lubricating oil production line. Reference Al-Najjar, B., and Alsyouf, I. (2004). Enhancing a company’s profit ability and competitiveness using integrated vibration-based maintenance: A case study, European Journal of Operational Research, 157(3): 643-657. Al-Najjar, B. and Algabroun, H. (2018), “Smart maintenance model using cyber physical systems” Diamond Jubilee National Convention of IIIE and International Conference ICIEIND 2018, India. Alev, A B., Caglayan, A., Ecem, M A. (2013). A cast study of effective factor on the right industrial lubricating oil choosing. Bilecik Seyh Edebali University, Chemical and Process Engineering, Bilecik, Turkey. Author profiles for this publication at: https://www.researchgate.net/publication/288004918. Ayad, S., Terrissa, L. S. and Zerhouni, N. (2018) ‘An IoT approach for a smart maintenance’, in 2018 International Conference on Advanced Systems and Electric Technologies, IC_ASET 2018. Institute of Electrical and Electronics Engineers Inc., pp. 210–214. Bokrantz, J. (2017), “Maintenance in digitalised manufacturing: Delphi-based scenarios for 2030”, International Journal of Production Economics, Vol. 191, pp. 154–169.29 Bokrantz, J., Skoogh, A., Berlin, C., Wuest, T., & Stahre, J. (2019a). Smart Maintenance: an empirically grounded conceptualization. International Journal of Production Economics, 107534.35. Bokrantz, J., Skoogh, A., Berlin, C., Wuest, T., & Stahre, J. (2019b). Smart Maintenance: a research agenda for industrial maintenance management. International Journal of Production Economics, 107547.38. Deloitte. (2015). Industry 4.0. Challenges and solutions for the digital transformation and use of exponential technologies. Djurdjanovica, D., Lee, J. and Ni, J. (2003),” Watchdog agent: an infotronics-based prognostics approach for product performance degradation assessment and prediction”, Advanced 16 Engineering Informatics, 17(3):109–125. Drath, R., & Horch, A. (2014). Industrie 4.0: Hit or hype? [Industry Forum]. IEEE Industrial Electronics Magazine, 8(2): 56–58. https://doi.org/10.1109/MIE.2014.231207919. Henson, C W. (2010). Model theory. Class Notes for Mathematics 571 Spring 2010. Mathematics Department University of Illinois 1409 West Green Street Urbana, Illinois 61801 email: henson@math.uiuc.edu www: http://www.math.uiuc.edu/~henson/. Kagermann, H., Helbig, J., Hellinger, A. and Wahlster, W., (2013). Recommendations for implementing the strategic initiative INDUSTRIE 4.0: Securing the future of German manufacturing industry; final report of the Industrie 4.0 Working Group. Forschungsunion. Kans, M., Galar, D. and Thaduri, A. (2016) ‘Maintenance 4.0 in Railway Transportation 27 Industry’, Proceedings of the 10th World Congress on Engineering Asset Management (WCEAM 28 2015), pp. 317–331. Karanovi, V V et al (2018). “Benefit of lubricant oil analysis for maintenance decision support”: a case study, Centre of Industrial Management, Celestijnenlaan 300A, 3001 Heverlee, Belgium OP Conf. Ser.: Mater. Sci. Eng. 393 012013. Kumar, P. and Singh, R. (2012). Neural Networks and Regression Modeling of Eco-friendly Melting Furnace Parameters using Biofuels. [Electronic version]. International Journal of Computer Applications, 43(1): 10-12. Labib, A. (2006). “Next-generation maintenance systems: towards the design of a self- maintenance machine”, paper presented at the IEEE International Conference on Industrial Informatics, pp. 213–217. Lee, J., Ghaffari, M. and Elmeligy, S. (2011), “Self-maintenance and engineering immune systems: towards smarter machines and manufacturing systems”, Annual Reviews in Control, 35(1): 111–122. Modh Harris bim, A J. (2012). PROG0101 Fundamentals of programming. FTMS College. Kuala Lumpur Malaysia. Micaecla, D., Gabrile, B., & Behnoush, D. (2018). Using field data for energy efficiency based on maintenance and operation optimization. Muhammed, S., and Smilia, M. (2014). A systematic literature review of software process improvement in small and medium web companies. Nadine, W., and Bernadelte, F. (2018). opportunities and limitation of software project management in geosciences and climate modeling. Neave, H. R. (1978), Statistics Tables for Mathematicians, Engineers, Economists and the Behavioural and Management Sciences. George Allen and Unwin Publishers Ltd. London, Pp 41-62. Niklaus, W. (2008). A brief history of software engineering. Olorunnishola, A.A.G and Omojogberun, Y.V (2019). Modeling of Melting Rate of an Hybrid Fuel-Fired Cupola Furnace, GSJ: 7(3): 273-283. Park, S. (2016). Development of Innovative Strategies for the Korean Manufacturing Industry by Use of the Connected Smart Factory (CSF). Procedia Computer Science, 91, 744– 750. Peter, P., David, Z., and Josef, B. (2019). Historical overview of maintenance management strategies: development from breakdown maintenance to predictive maintenance in accordance with four industrial revolutions. Qiao, G. and Weiss, B. A. (2016) ‘Advancing Measurement Science to Assess Monitoring, Diagnostics, and Prognostics for Manufacturing Robotics’, Int J Progn Health Manag., 7. Su-Hua, W., Durgesh, S., Dengie, C. (2011). software development and quality problem solution by TRIZ. (procedural computer science 5 (2011) 730–735). Thoben, K. D., Wiesner, S. A., & Wuest, T. (2017). “Industrie 4.0” and smart manufacturing- a review of research issues and application examples. In International Journal of Automation Technology, 11(1): 4-16. Ungureanu, U., Sisak, F., kristaly, M D., &Moraru, S. (2005). Simulstion modeling input & data collection and analysis Keywords - Production rate, Energy consumption rate, equipment breakdowns, maintenance quality |