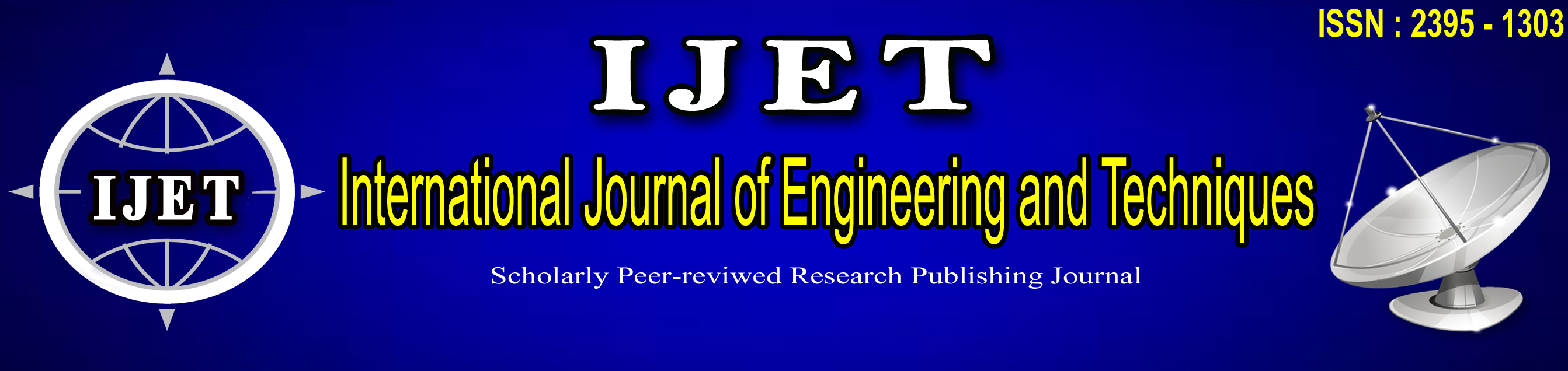
Submit your paper : editorIJETjournal@gmail.com ![]() ISSN : 2395-1303 Year of Publication : 2022 ![]() ![]() ![]() MLA Style: -Christian Yonathan S, Imam Halim Mursyidin, Dede Irawan, Doni Prasetyo , Prediction of Students Graduation Rate Using Mapreduce " " Volume 8 - Issue 1 January - February,2022 International Journal of Engineering and Techniques (IJET) ,ISSN:2395-1303 , www.ijetjournal.org APA Style: - Christian Yonathan S, Imam Halim Mursyidin, Dede Irawan, Doni Prasetyo " Prediction of Students Graduation Rate Using Mapreduce " Volume 8 - Issue 1 January - February,2022 International Journal of Engineering and Techniques (IJET) ,ISSN:2395-1303 , www.ijetjournal.org Abstract Colleges are now in competitive competition. Every college tries to improve academic quality and accreditation. One of the accreditation assessments of tertiary institutions is that students graduate on time, with criteria for passing fast, graduating right, passing late, and dropping out. This research was conducted using a classification method that was applied to predict the final grade of students. This study proposes the K-Nearest Neighbor (K-NN) algorithm with the most important attribute is GPA for each semester. In this study, using Hadoop-MapReduce tools with data selection contained in the Forlap Dikti Indonesia, for students Budi Luhur University Jakarta in 2009-2011. The information generated will display the graduation rate of students, from this research the academic side can make decisions regarding higher education policies Reference [1] Kong Xiangsheng, “Big Data X-Learning Resources Integration and Processing in Cloud Environments”, International Journal of Emerging Technologies in Learning (iJET), Universität Kassel, Germany, 2014, pp. 22-26. [2] Xiaoling Huang,Yangbing Xu, Shuai Zhang and Wenyu Zhang, “Association Rule Mining for Selecting Proper Students to Take Part in Proper Discipline Competition: A Case Study of Zhejiang University of Finance and Economics”, International Journal of Emerging Technologies in Learning (iJET), Universität Kassel, Germany, 2018, pp. 100-113. [3] Argelia Berenice Urbina Najera and Jorge de la Calleja Mora, “Brief Review of Educational Applications Using Data Mining and Machine Learning”, Revista Electrónica de Investigación Educativa, Universidad Autónoma de Baja California, Mexico, 2017, pp. 84-96. [4] Pavan B M and K. Thippeswamy, “A New Approach of Analysis of Student Results by Using MapReduce”, International Research Journal of Engineering and Technology (IRJET), VTU Centre for PG Studies, Mysuru, Karnataka, 2017, pp. 1892-1895. [5] Diptimayee Baliarsingh and Samiksha Hemant Parab, “Analysis of student academics performance using Hadoop”, International Journal of Advance Research, Ideas and Innovations in Technology, Universität Kassel, Germany, 2018, pp. 4-16. [6] Haixia Lu and Jinsong Yuan, “Student Performance Prediction Model Based on Discriminative Feature Selection”, International Journal of Emerging Technologies in Learning (iJET), Universität Kassel, Germany, 2018, pp. 55-68. [7] Yuan Zhang and Wnbo Jiang, “Score Prediction Model of MOOCs Learners Based on Neural Network”, International Journal of Emerging Technologies in Learning (iJET), Universität Kassel, Germany, 2018, pp. 171-182. [8] Feng-juan Liu, “A Quantitative Evaluation Model of Interactive Whiteboard Classroom Based on K-Means Algorithm”, International Journal of Emerging Technologies in Learning (iJET), Universität Kassel, Germany, 2018, pp. 89-101. [9] Irina Zheliazkova, Oktay Kir and Adriana Borodzhieva, “Knowledge Prediction of Different Students’ Categories Trough an Intelligent Testing”, TEM Journal, UIKTEN, Serbia, 2015, pp. 44-53. [10] Marian Bucos and Bogdan Dragulescu, “Predicting Student Success Using Data Generated in Traditional Educational Environments”, TEM Journal, UIKTEN, Serbia, 2018, pp. 617-625. [11] Husnul Rahmawati Sakinnah, Adhistya Erna Permanasari and Indah Soesanti, “Classification of Metacognitive into Two Catagories to Support the Learning Process”, Jurnal Pendidikan Sains, Universitas Negeri Malang, Indonesia, 2017, pp. 11-16. [12] Jianhui Chen and Jing Zhao, “An Educational Data Mining Model for Supervision of Network Learning Process”, International Journal of Emerging Technologies in Learning (iJET), Universität Kassel, Germany, 2018, pp. 67-77. [13] Anthony Mark Langan, Peter Dunleavy and Alan Fielding, “Applying Models to National Surveys of Undergraduate Science Students: What Affects Ratings of Satisfaction?”, Education Sciences, MDPI AG, Switzerland, 2013, pp. 193-207. [14] Preston A. Clark and Mingyuan Zhang, “The Impact of Teacher Technology Training on Student Technology Assessments”, Journal of Research in Science, Mathematics and Technology Education, Ferhat Ozturk, United States, 2018, pp. 229-266. [15] Anjad Abu Saa, “Educational Data Mining & Students’ Performance Prediction”, (IJACSA) International Journal of Advanced Computer Science and Applications, Ajman University, United Arab Emirates, 2016, pp. 212-220 Keywords -— Hadoop, Mapreduce, KNN, Graduation, Student |