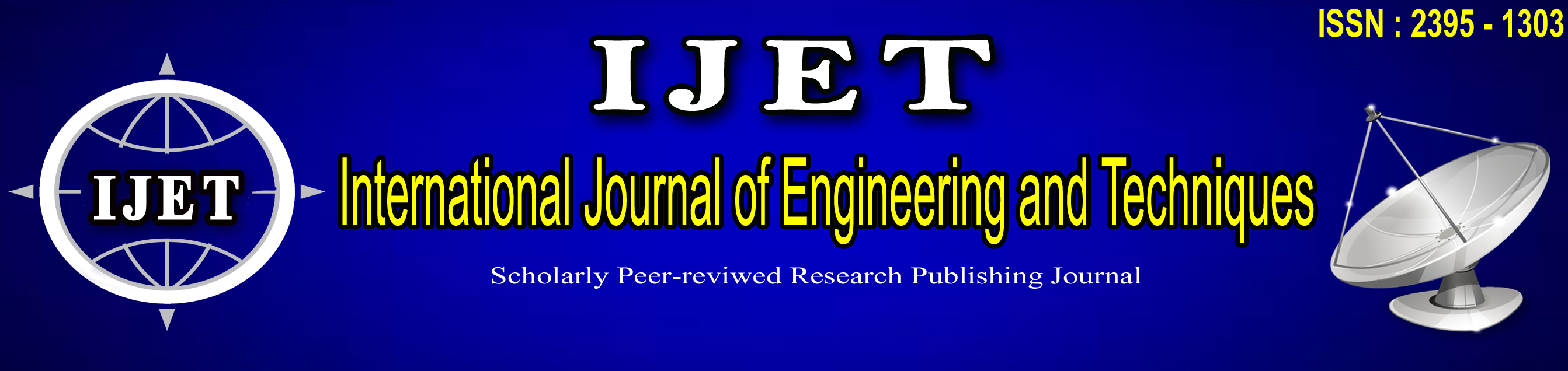
Submit your paper : editorIJETjournal@gmail.com ![]() ISSN : 2395-1303 Year of Publication : 2021 ![]() ![]() ![]() MLA Style: - Ms. Shubhangi Pimpalzare, Dr. S S Mungona , Different Wavelets For Medical Image Compression In Telemedicine’s " " Volume 7 - Issue 5 September - October,2021 International Journal of Engineering and Techniques (IJET) ,ISSN:2395-1303 , www.ijetjournal.org APA Style: - Ms. Shubhangi Pimpalzare, Dr. S S Mungona " Different Wavelets For Medical Image Compression In Telemedicine’s " Volume 7 - Issue 5 September - October,2021 International Journal of Engineering and Techniques (IJET) ,ISSN:2395-1303 , www.ijetjournal.org Abstract - Transferring medical images from one center to another is common use in telemedicine. These high-quality images stored in DICOM format require higher bandwidth in transmission and large storage space in PACS (Picture Archieving and Communication System) memory. Therefore reducing the image size for preserving diagnostic information has become a need. In this sense, medical image compression is technique that overcomes both transmission and storage cost by lossy and lossless compression algorithms. There are numerous compression methods developed for region-based studies generally used in radiography, computed tomography (CT) and magnetic resonance images (MRI). The use of digital medical images is increases very fast. Medical images like CT scan, ultrasound, dental X-ray etc, require large amounts of memory storage. Even to transmit an image over a wireless or LAN network could take more time. Due to this reason medical image compression is important. Related to medical images many compression methods are available. However, the lossless ( for diagnostic and legal reasons) techniques, which allows for perfect reconstruction of original image, yield compression rates of at most 2 only, while the techniques that yields higher compression rates are lossy. To meet this challenge, we have developed a hybrid compression schemes which is diagnostically lossless with good compression ratio. Due to its simplicity the hardware realization is also easy and not cost effective compare to JPEG method. Along with that, very limited researchers take a challenge to apply hardware on their implementation. Referring to the previous work reviewed, most of the compression method used lossless rather than lossy. For implementation using software, MATLAB is the famous candidates among researchers. In term of analysis, most of the previous works conducted objective test compared with subjective test. This paper thoroughly reviews the recent advances in medical image compression mainly in terms of types of compression, software and hardware implementations and performance evaluation. Furthermore, challenges and open research issues are discussed in order to provide perspectives for future potential research. In conclusion, the overall picture of the image processing landscape, where several researchers more focused on software implementation and various combination of software implementation. Reference 1.J. Satheeshkumar, S. Arumugaperumal, R. Rajesh, C. Kesavdas (2011), „On Experimenting with Single Subject Multi-Session fMRI Analysis of Human Brain‟, International Journal of Wisdom based Computing, vol. 1(1) page 23-34. 2. P. V. Peck, “New medical imaging technology,” in Proc. Special Symp. Maturing Technologies and Emerging Horizons in Biomedical Engineering,Nov. 1988, pp. 113–114. 3. Cherry SR: Fundamentals of positron emission tomography. Journal of Clinical Pharmacology. 2001; 41: 482-491. 4. Rossyln VA, DICOM (Digital Imaging and Communications in Medicine), PS 3.5 Data Structures and Encoding, NEMA, 1999. 5.Wallace, G.K. (1991) „The JPEG still picture compression standard‟, Communications of the ACM,Vol. 34, No. 4, April, pp.30–44. 6.DeVore, R.A., orn Jawerth, B. and Lucier, B.J. (1992) „Image compression through wavelet transform coding‟, IEEE Transactions on Information Theory, March, pp.719– 746. 7. Matthew J. Zukoski, Terrance Boult , Tunc Iyriboz , „A novel approach to medical image compression‟, Int. J. Bioinformatics Research and Applications, Vol. 2, No. 1, 2006. 8. Michael W. Marcellin, Michael J. Gormish, Ali Bilgin, Martin P. Boliek (2000), „An Overview of JPEG2000‟, Proc. of IEEE Data Compression Conference, pp. 523-541. 9. Rafael C Gonzalez, Richard E Woods,Digital Image Processing, 3rd edition, Publisher: Pearson, 2008. 10. A. Nait-Ali and C. Cavaro-Menard, Eds., „Compression of Biomedical Images and Signals‟, ISTE / WILEY, 2008. 11. H. K. Huang, PACS, Cambridge, U.K.: VCH , 1996. 12. Kivijärvia, J., Ojalaa, T., Kaukorantaa, T., Kubab, A., Nyulb, L. and Nevalainena, O. (1999) „A comparison of lossless compression methods for medical images‟, IEEE Data Compression Conference, pp.718– 721 13..D. Taubman and M.W. Marcellin, JPEG2000: Image Compression Fundamentals,Standard and Practice, 1st ed. Norwell, MA: Kluwer Academic,2002. 14.Koff, D.A. and Shulman, H. (2006) „An overview of digital compression of medical images. Canwe use lossy image compression in radiology?‟, Canadian Association of Radiologists Journal, Vol. 57, No. 4, pp.211–217. 15. Agarwal, A. Rowberg, A.H. Yongmin Kim (2003), „Fast JPEG 2000 decoder and its use in medical imagin‟, IEEE Transactions on Information Technology in Biomedicine, vol. 7, 16. Gonzalez RC, Woods RE (2002) Digital Image Processing. (2nd Edition), Prentice Hall, Upper Saddle River, New Jersey, USA, pp. 409-518. 17. Sophia P, Anitha J (2014) Implementation of Region Based Medical Image Compression for Telemedicine Application. International Conference on Computational Intelligence and Computing Research (ICCIC), India. 18. Raja JA, Raja G, Khan K (2013) Selective Compression of Medical Images using Multiple Regions of Interest. Life Science Journal 10(9): 394-397. 19.Seddiki A, Guerchi D (2014) Medical Image Compression by Region of Interest based on SPIHT and Global Thresholding using Huffman Coding. Recent Advances in Electrical Engineering and Educational Technologies, Greece. 20.Kaur A, Goyal M (2014) ROI Based Image Compression of Medical Images. International Journal of Computer Science Trends and Technology (IJCST) 2(5): 162-166. 21. Anandan A, Sabeenian RS (2016) Medical Image Compression Using Wrapping Based Fast Discrete Curvelet Transform and Arithmetic Coding. Circuits and Systems 7(8): 2059-2069 Keywords - Matlab, MSE(mean square error);PSNR(peak signal to noise ratio);COC(correlation coefficient);Huffman coding ;CT scan images; Ultrasound images; Dental X- ray |