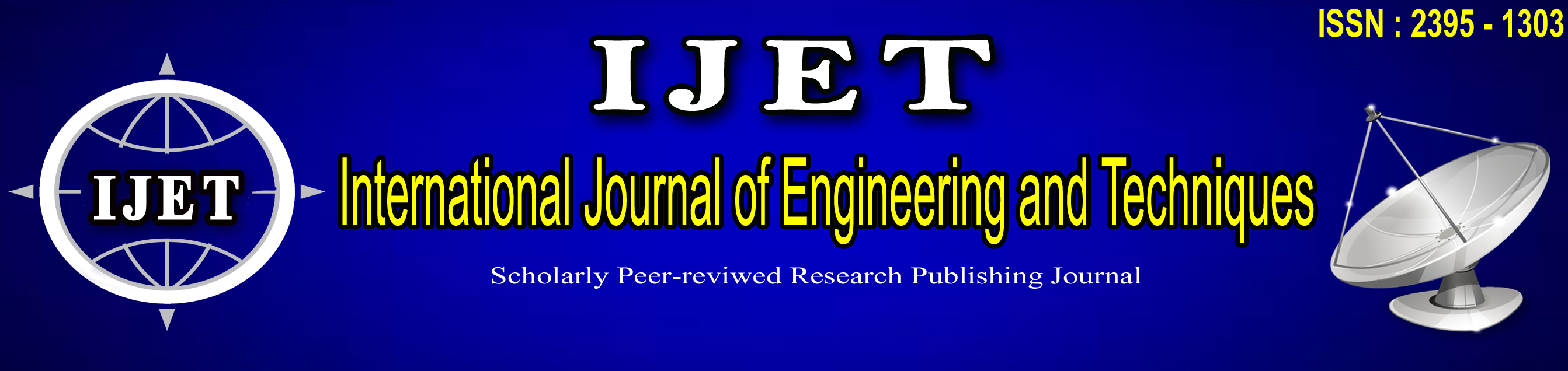
Submit your paper : editorIJETjournal@gmail.com ![]() ISSN : 2395-1303 Year of Publication : 2021 ![]() ![]() ![]() MLA Style: -Gaddam Akhil Reddy, Kothapally Nithesh Reddy,Dr. Vijayalakshmi Kakulapati , Exploration and Assessment of Multiple Crowd Sourced Feedbacks " " Volume 7 - Issue 5 September - October,2021 International Journal of Engineering and Techniques (IJET) ,ISSN:2395-1303 , www.ijetjournal.org APA Style: - Gaddam Akhil Reddy, Kothapally Nithesh Reddy,Dr. Vijayalakshmi Kakulapati " Exploration and Assessment of Multiple Crowd Sourced Feedbacks " Volume 7 - Issue 5 September - October,2021 International Journal of Engineering and Techniques (IJET) ,ISSN:2395-1303 , www.ijetjournal.org Abstract The majority of moviegoers use websites like IMDb, Amazon, and Yelp to research and select their next viewing or purchase. Currently, moviegoers make their choices based on IMDb or Amazon ratings and reviews of films they've seen. As outlined in this article, a better technique is proposed: the subjective content of movie critics' movie scores and critiques may be evaluated and projected onto a picture, resulting in an emotional map. The second step is to seek films with emotion maps that have certain emotion map patterns that appeal to you. Concerning decision-making processes, sentiment analysis has long been a point of concern for marketing, business, and management departments. When we conduct sentiment analysis, we're looking for emotions and points of view in a piece of writing. It identifies and validates a person's feelings about a certain piece of material for the reader. Product reviews, blogs, status updates, and tweets, among other things, are all examples of sentiment data seen on social media sites. This massively generated data may be used for sentiment analysis to express the opinions of the general public on products. This article presents a highly accurate sentiment analysis algorithm for Amazon, IMDB, and Yelp product, movie, and restaurant reviews. These reviews are classified into two categories: positive and negative, and we employ a variety of classifiers to determine which category is more favorable. The best classifier is chosen based on how accurate it is. Reference 1. Boya Yu, Jiaxu Zhou, Yi Zhang, Yunong Cao, “Identifying Restaurant Features via Sentiment Analysis on Yelp Reviews”, arxiv,20 Sep 2017 2. Filieri, R.; Raguseo, E.; Vitari, C. When are extreme ratings more helpful? Empirical evidence on the moderating effects of review characteristics and product type. Comput. Hum. Behav. 2018, 88, 134–142. 3. Huang, A.H.; Chen, K.; Yen, D.C.; Tran, T.P. A study of factors that contribute to online review helpfulness.Comput. Hum. Behav. 2015, 48, 17–27. 4. Pantelidis, I.S. Electronic meal experience: A content analysis of online restaurant comments. Cornell Hosp. Q.2010, 51, 483–491. 5. P. Sasikala, L.M. I Sheela,“Sentiment Analysis of Online Food Reviews using Customer Ratings”, International Journal of Pure and Applied Mathematics, Volume 119 No. 15, 3509-3514,2018. 6. Yan, X.; Wang, J.; Chau, M. Customer revisit intention to restaurants: Evidence from online reviews. Inf. Syst Front. 2015, 17, 645–657 7. .Tri Doan, Jugal Kalita, ‘‘Sentiment Analysis of Restaurant Reviews on Yelp with Incremental Learning”, 2016 15th IEEE International Conference on Machine Learning and Applications. 8. Gao, B.; Hu, N.; Bose, I. Follow the herd or be myself? An analysis of consistency in behavior of reviewers and the helpfulness of their reviews. Decis. Support Syst. 2017, 95, 1–11 . 9. Chua, A.Y.; Banerjee, S. Helpfulness of user-generated reviews as a function of review sentiment, product type and information quality. Comput. Hum. Behav. 2016, 54, 547–554. 10. Ma, Y.; Xiang, Z.; Du, Q.; Fan, W. Effects of user-provided photos on hotel review helpfulness: An analytical approach with deep learning. Int. J. Hosp. Manag. 2018, 71, 120–131. 11. Nam, S.; Ha, C.; Lee, H. Redesigning In-Flight Service with Service Blueprint Based on Text Analysis. Sustainability 2018, 10, 4492. 12. L. Garcia-Moya, H. Anaya-Sanchez, and R. Berlanga-Llavori, ‘‘Retrieving product features and opinions from customer reviews,’’ IEEE Intell. Syst.,vol. 28, no. 3, pp. 19–27, May 2013. 13. R. Jing, Y. Yu, and Z. Lin, ‘‘How service-related factors affect the survival of B2T providers: A sentiment analysis approach,’’ J.Organizational Comput. Electron. Commerce, vol. 25, no. 3, pp. 316– 336, Jul. 2015. 14. Ł. Augustyniak, P. Szymański, T. Kajdanowicz, and W. Tuligłowicz, ‘‘Comprehensive study on lexicon-based ensemble classification sentiment analysis,’’ Entropy, vol. 18, no. 1, p. 4, Dec. 2015. 15. D.K.Kirange and 2Dr. Ratnadeep R. Deshmukh, “Aspect and Emotion Classification of Restaurant and Laptop Reviews Using Svm”, International Journal of Current Research Volume. 8, Ise-03, pp. 28352- 28356, March, 2016. 16. Wang, X.; Tang, L.R.; Kim, E. More than words: Do emotional content and linguistic style matching matter on restaurant review helpfulness? Int. J. Hosp. Manag. 2019, 77, 438–447 17. Hu, Y.H.; Chen, K.; Lee, P.J. The effect of user-controllable filters on the prediction of online hotel reviews.Inf. Manag. 2017, 54, 728–744. 18. A.-M. Popescu and O. Etzioni, ‘‘Extracting product features and opinions from reviews,’’ in Proc. Conf. Human Lang. Technol. Empirical Methods Natural Lang. Process. (HLT), Stroudsburg, PA, USA: Association for Computational Linguistics, 2005, pp. 339–346, doi: 10.3115/1220575.1220618 Keywords -Opinion Mining, SVM, Logistic Regression, Lexicon, Pipeline, TF-IDF Vectorizer, Ensemble Learning |