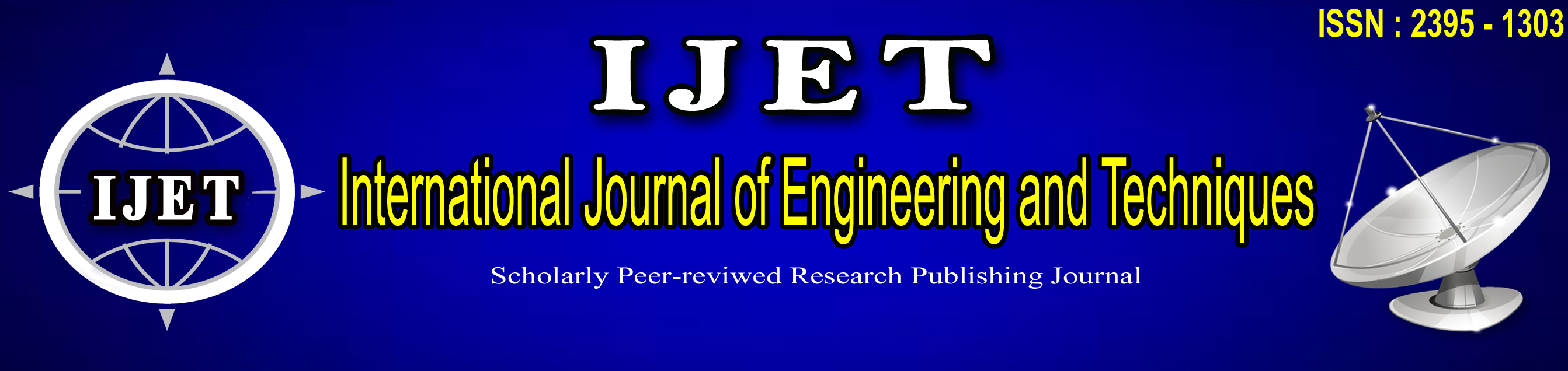
Submit your paper : editorIJETjournal@gmail.com ![]() ISSN : 2395-1303 Year of Publication : 2020 ![]() ![]() ![]() MLA Style: Mr.S.Sambasivam, M.C.A., M.Phil, Mr. S. Mohanasundaram, M C A MODEST AND TARGET CONSCIOUS E-SCIENCE WORKFLOW PLANNING IN CLOUDS " Volume 6 - Issue 2(1-5) March - April,2020 International Journal of Engineering and Techniques (IJET) ,ISSN:2395-1303 , www.ijetjournal.org APA Style: Mr.S.Sambasivam, M.C.A., M.Phil, Mr. S. Mohanasundaram, M C A MODEST AND TARGET CONSCIOUS E-SCIENCE WORKFLOW PLANNING IN CLOUDS " Volume 6 - Issue 2(1-5) March - April,2020 International Journal of Engineering and Techniques (IJET) ,ISSN:2395-1303 , www.ijetjournal.org Abstract Cloud computing is rapidly developing and lots of extra cloud carriers are emerging. Cost efficiency and resource price maximization turn out to be two major worries of cloud providers to remain aggressive while making profit. The income maximization problem in federated cloud environments co-operate to increase the degree of multiplexing has been investigated. Outline novel economics-inspired aid allocation mechanisms to address the income maximization hassle from the attitude of a cloud issuer performing solely. Admission manage mechanisms tailored within a Profit management framework to maximize resource value has been proposed. Existing abstractions for in-memory garage on clusters, such as dispensed shared memory, key value stores, databases, and Piccolo, offer an interface primarily based on fine-grained updates to mutable state (e.g., cells in a table). Our resource allocation technique is primarily based on I find many danger in EDPP on a couple of clouds. It design a aid discovery protocol, specifically Event handler E-DAG(Direct Acrylic Graph), to locate those certified nodes. It chooses EDPP-E-DAG as the DHT overlay to evolve to the multidimensional feature. Some of them are inherit within the process of planning like pressure and different arise because of shortcoming of the techniques on multi cloud by means of themselves on this proposed work. With this interface, the handiest methods to offer fault tolerance are to replicate the statistics across machines or to log updates across machines. Where various pricing plans in multiple marketplaces are supported via the provider an auction-based dynamic pricing mechanism suitable for selling the spare ability of the records center. A consciousness of the proposed dynamic pricing mechanism within a pricing as a service framework. Cost powerful resource allocation based totally on following techniques are Cost Efficiency of the Cloud: Cost reductions and profit increases, Pay as it cross pricing, Implications of multi Scheduling and resource allocation as a fee efficient solution: Exploitation of utility characteristics, explicit consideration of user experience/satisfaction. Reference [1] Rakesh Agrawal Jerry Kiernan et al, “Skeleton pruning by contour partitioning with discrete curve evolution,” IEEE Trans. Pattern Anal. Mach. In tell vol. 29, no. 3, pp. 449–462, Mar. 2004. [2] Claudio Lucchese et al, “A weighted finite-state framework for correcting errors in natural scene OCR,” in Proc. 9th Int. Conf. Document Anal. Recognit. Sep. 2001, pp. 889–893. [3] Vıctor R. Doncel, Nikos et al, “Automatic detection and recogniton of signs from natural scenes,” IEEE Trans. Image Process., vol. 13, no. 1, pp. 87–99, Jan. 2000. [4] A. Coates et al., “Text detection and character recognition in scene images with unsupervised feature learning,” in Proc. ICDAR, Sep. 2011, pp. 440–445. [5] N. Dalal and B. Triggs, “Histograms of oriented gradients for human detection,” in Proc. IEEE Conf. Comput. Vis. Pattern Recognit., Jun. 2005, pp. 886–893. [6] T. de Campos, B. Babu, and M. Varma, “Character recognition in natural images,” in Proc. VISAPP, 2009. [7] B. Epshtein, E. Ofek, and Y. Wexler, “Detecting text in natural scenes with stroke width transform,” in Proc. CVPR, Jun. 2010, pp. 2963–2970. [8] P. F. Felzenszwalb, R. B. Girshick, D. McAlester, and D. Ramanan, “Object detection with discriminatively trained part-based models,” IEEE Trans. Pattern Anal. Mach. In tell, vol. 32, no. 9, pp. 1627–1645, Sep. 2010. [9] Rakesh Agrawal and Jerry Kiernan et al , “Learning shape prior models for object matching,” in Proc. CVPR, Jun. 2006, pp. 848–855. [10] N. F. Johnson, Z. Duric, “Text extraction and document image segmentation using matched wavelets and MRF model,” IEEE Trans. Image Process., vol. 16, no. 8, pp. 2117– 2128, Aug. 2000. Keywords Scientific Workflow, Scheduling, Budget, Deadline, Cloud. |